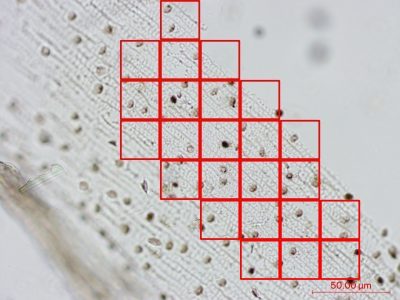
A new study shows that it is possible to automate the detection and classification of phytoliths with a high-level of accuracy, up to a species level.
This method has the potential to allow the development of much larger analytical datasets in a fraction of the time than was previously feasible, as well as to assure consistency in phytolith identification and increase the validity of sample analysis.
The incorporation of Machine Learning-based workflows in archaeology, while still little explored beyond site detection studies (Berganzo-Besga et al., 2021; Orengo et al., 2021), presents significant potential within archaeological research.
The doctoral researcher Iban Berganzo-Besga (GIAP research group at the ICAC) led by Dr. Hèctor A. Orengo (ICAC) and Dr Felipe Lumbreras (Computer Vision Center, UAB), in collaboration with Dr Monica N. Ramsey (University of Toronto Mississauga; McDonald Institute for Archaeological Research, University of Cambridge) and Paloma Aliende (ICAC), has developed a Deep Learning (DL) algorithm for the automated detection and classification of multi-cell phytoliths.
Automated detection and classification of multi-cell Phytoliths using Deep Learning-Based Algorithms. Iban Berganzo-Besga, Hèctor A. Orengo, Felipe Lumbrera, Paloma Aliende, Monica N.Ramsey. Journal of Archaeological Science, 2022, 105654, ISSN 0305-4403, https://doi.org/10.1016/j.jas.2022.105654.
The use of DL algorithms has the potential to provide tools for the automated identification of phytoliths.
Multi-cell phytoliths, particularly grass husks, provide more specific genera level identifications and are therefore critical to the archaeological application of phytolith analysis (Rosen, 1992). Also, given the complexity of forms that multi-cells present, and the similarity between these forms, these identifications can be time consuming and challenging even for experienced phytolith analysts. The use of DL algorithms has the potential to provide tools for the automated identification of phytoliths. This approach has been tested using three key phytolith genera for the study of agricultural origins in Near Eastern archaeology: Avena, Hordeum and Triticum.
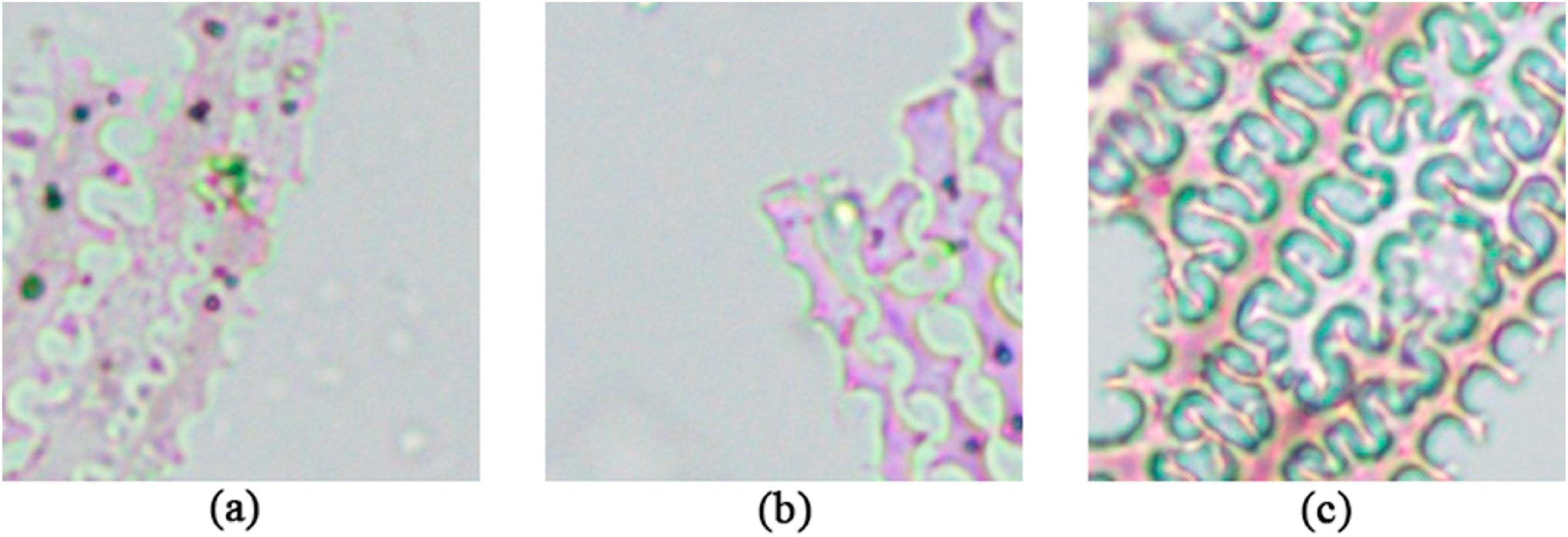
The method and algorithm published in the Journal of Archaeological Science, has been able to identify and classify the three genera with more than 93% overall confidence and two species (Triticum boeoticum Acc. and Triticum dicoccoides Acc.) with a 100% confidence.
Complex digital microscopes can incorporate DL algorithms, allowing near-instantaneous automatic phytolith-type counts, a radical improvement on the current analysis speeds. Beside this, the algorithm is designed to be employed by other interested parties using freely available computational resources such as Google Colaboratory.
The published paper can provide an important methodological tool for researchers using phytoliths in vegetation history, archaeobotany, palaeoecology, human environmental-interactions and the origins of agriculture.
This method has the potential to revolutionise all these fields by allowing not just the development of much larger analytical datasets in a fraction of the time than was previously feasible but also by allowing the incorporation of new measurements and analysis methods (such as fragmentation patterns, phytolith size, etc.), ensuring consistency in phytolith identification, and increasing the validity of sample analysis by moving from statistical estimations to total phytolith counts.
The incorporation of new methods and automated detection and classification algorithms should ultimately allow archaeologists to concentrate their efforts into the historical and sociocultural interpretations that make archaeological insight unique and necessary.
Contributions by authors
- Iban Berganzo-Besga: formal analysis, investigation, methodology, validation, software, data curation, writing of the original draft, visualisation.
- Felipe Lumbreras: methodology, resources, writing, review and editing, supervision.
- Monica N. Ramsey: conceptualisation, data curation, writing of the original draft, project administration, funding acquisition.
- Hèctor A. Orengo: conceptualisation, methodology, re- sources, writing, review and editing, supervision, project administration, funding acquisition.
- Paloma Aliende: data curation.
Funding:
Monica N. Ramsey is a Leverhulme Early Career Fellow (EFC-2020-318) and was awarded a D M McDonald Research Grant from the McDonald Institute for Archaeological Research (Deep Origins: AI Deep Learning ID of Plant Phytoliths for the Origins of Agriculture) which partly funded I.B–B’s analysis. Hèctor A. Orengo is a Ramón y Cajal Fellow (RYC-2016-19637) of the Spanish Ministry of Science, Innovation and Universities. Felipe Lumbreras work is supported in part by the Spanish Ministry of Science and Innovation project BOSSS TIN2017-89723-P. Some of the GPUs used in these experiments are a donation of Nvidia Hardware Grant Programme.
Bibliography
Berganzo-Besga, I.; Orengo, H. A.; Lumbreras, F.; Carrero-Pazos, M.; Font, J.; Vilas-Estévez, B. “Hybrid MSRM-Based Deep Learning and Multitemporal Sentinel 2-Based Machine Learning Algorithm Detects Near 10k Archaeological Tumuli in North-Western Iberia”. Remot Sens 2021, 13, 4181. https://doi.org/10.3390/rs13204181
Orengo, H. A.; Garcia- Molsosa, A.; Iban Berganzo-Besga; Landauer, J.; Aliende, P.; Tres-Martínez, S. “New developments in drone-based automated surface survey: Towards a functional and effective survey system”. Arcaheol. Prospect 2021, 1–8. https://doi.org/10.1002/arp.1822
Rosen, A. M. “Preliminary Identification of Silica Skeletons from Near Eastern Archaeological Sites: An Anatomical Approach”. In: Rapp, G. R.; Mulholland, S. C. (eds.), Phytolith Systematics: Emerging Issues. Advances in Archaeological and Museum Science, 1a ed., Springer: Boston (EUA), 1992; Vol. 1, p. 129-147. https://doi.org/10.1007/978-1-4899-1155-1_7
The Catalan Institute of Classical Archaeology (ICAC) is a CERCA center created as a consortium in 2002 by the Generalitat de Catalunya (Catalan government) and the University Rovira i Virgili. It is based in Tarragona, a city recognized as a World Heritage Site by UNESCO in 2000 and specializes in advanced research and training in classical archaeology. More information: www.icac.cat/en
The Computer Vision Center (CVC) is a CERCA center founded in 1995 as a consortium formed by the Generalitat de Catalunya and the Autonomous University of Barcelona (UAB). With an outstanding team of more than 130 researchers and technicians, CVC’s mission is to develop prestigious research with international impact in the field of Computer Vision, transfer knowledge to companies and society, and train scientists and high-level professionals. More information: www.cvc.uab.es
The Department of Anthropology at the University of Toronto Mississauga is dedicated to disseminating anthropological knowledge through research, teaching, writing, and other forms of outreach. The research expertise of our faculty spans a broad spectrum of activities and geographic regions, covering both the Sciences and Social Sciences. Our exceptional faculty include distinguished scholars with international reputations, who are very active in research, while continuing to provide excellent training and learning opportunities for students. More information: https://www.utm.utoronto.ca/anthropology/